Artificial intelligence is already replacing workers and can do all sorts of things such as virtual personal assistance, image recognition, voice recognition and even driving cars. Some people find this transformation exciting whilst others are fearful, predicting an existential crisis where humans are no longer required.
But I’m cautiously optimistic because Recruitment Genius has experienced some benefits in machine learning. So, in this article, I’d like to share some of my insights with you.
We all have relative strengths and weaknesses
Machines have many strengths that in some ways are superior to humans – your humble calculator can outperform you. Equally, humans have stronger inter-personal skills and creativity. We are able to transfer ideas from one domain to another – something machines can’t rival, yet.
Machines also have a weakness because they are ‘narrow thinkers’. For example, a machine may be victorious at chess but can’t win at chequers. Additionally, machines don’t have general intelligence and common sense like humans do. For instance, a machine can read Latin but this doesn’t mean it knows where the phrase “Achilles heel” came from.
But neither machines or humans are perfect, we both make mistakes. Facial recognition software has a 3-4% error rate compared to humans with an average 5% error rate (when bumping into people at conferences, I’d estimate mine is far worse!).
We are also both biased. A lot of psychometric assessments are biased because the sample group were from a limited demographic which skews the results. Equally humans are biased because we like people that are like ourselves (aka, the Halo & Horns effect).
Combining these points of making mistakes and bias in a recent fun example was an automated chatbot in China. When asked “do you like the communist party?” it was met with a defiant “No”. This case illustrates the fact that machines sometimes get it wrong because they are embedded with the views of rogue/unpatriotic humans.
Artificial intelligence is improving
The reason people are becoming so excited about recent improvements is because of the way machines are learning. In the past humans had to fully understand a task and programme software (such as writing calculations in Excel). But now machines learn on their own because we provide examples of a desired outcome. Meaning machines can programme themselves.
This creates a new issue because ‘black boxes’ can rarely explain their decisions and behaviour even to their developers. But it’s important to understand their judgement so we can trust them and detect flaws. Like when a Tesla driver was killed using autopilot because the computer failed to spot a white truck against the background of a bright blue sky.
Naturally the speed of machine learning is not approaching human-level intelligence; it only takes a few instances for a baby to recognise its mother’s face.
All the learning potential is now possible for three reasons:
- Computers have significantly more power so systems can be trained quicker
- There has been an explosion in data required to train software. Most important of these are image and voice recognition. But with additional data from the Internet of Things (IOT) there will be more possibilities
- Improvements have been made to the algorithms we use
What tends to hold us back is the creative ways to utilise artificial intelligence to create more value. However, it won’t necessarily require us to solve seemingly big problems because often a simple solution has compound benefits.
Artificial intelligence is already being used at Recruitment Genius
If you’re thinking machine learning is too futuristic, I’d like to give you a home-grown example of how Recruitment Genius’s customers benefit from it today.
When we started the company in 2009 a lot of administrators would painstakingly review every CV to see how relevant it was for an employer’s job. Unfortunately, employees would not always be able to make objective decisions because they brought their personal bias to work (something we all have).
Naturally staff also became quickly fatigued when conducting this repetitive task which could impair their judgement. There is lots of evidence that fatigue effects judgement; for example judges give more punitive decisions as the day goes on.
For these reasons, we experimented with machine learning. Fortunately our employees and customers had already tagged millions of records, indicating which applicants were successful and which weren’t. Therefore we could create a ‘supervised’ machine learning experiment. Importantly, the dataset we had was so broad that we didn’t need to be concerned about introducing an implicit bias.
Machines began learning by creating experiments and comparing against the benchmark set by our employees and customers. After a lot of effort on behalf of data scientists and machines our CV Score was born, statistically able to predict how relevant a job application is.
There have been many benefits:
- Employers have an indication of how much time to spend reviewing an applicant
- We have an effective and efficient process that we will continue to benefit from for the foreseeable future since the task is not going to fundamentally change
- Our staff are no longer fatigued by a repetitive task and have been upskilled to add more value to the business
Artificial intelligence is already effecting jobs today but might create new jobs
Machine learning is already encroaching on certain human tasks. For this reason, ‘narrow’ and repetitive tasks are no longer going to be a big source of employment. More worrying for workers, we are only scratching the surface as to how roles can be chunked into ‘narrow’ tasks. This is why up to 15 million jobs in the UK could be automated over the coming decades, and up to 30% of jobs in the UK may be automated by the 2030s.
These problems are going to be even worse in emerging economies that rely on mopping up repetitive tasks with cheap labour. For instance, sewing a t-shirt used to be complex because of the flimsy material. But robots using artificial intelligence have cracked it meaning organisations no longer need to place large orders with cheap overseas providers. Instead they can stitch on demand.
Another reason emerging economies will struggle to benefit from artificial intelligence is because they don’t have access to as many datasets because they are not digitally-driven.
But don’t think it’s only low-value tasks that will be replaced by machines. Expensive financial analysts are competing against Quant investment algorithms. It’s easier to replace the work of a Chartered Accountant than a cleaner.
Education will also be an important factor. Workers educated to secondary level are 15 times more likely to be automated than those with a PhD or Master’s degree (source: Arntz M, Gregory T, Zierahn U. 2016, The risk of automation for jobs in OECD countries).
Despite this I believe the future will combine the strengths of both humans and machines to be the most effective. This is particularly important for risky decisions because machines can get things wrong (aka false-positives). For example, software diagnosing cancerous cells can get it wrong and so needs to send obscure examples for human verification. This means humans will become more effective and productive because they are supported by technology.
Almost no sector will be immune from artificial intelligence and it will affect industries in many different ways. For example:
- Healthcare will have more accurate diagnosis
- Pharmaceuticals can use genetic studies
- Education can support learners by delivering more personalised learning plans
- Logistics can benefit from less accidents and efficient traffic flows
- Public services can be more responsive to consumption of public services
- Finance is already making investment decisions
- Legal sector can support high volume low value cases with chatbot-style interactions – in effect automating some junior lawyer tasks
- Retail can personalise products and make better recommendations
Artificial intelligence will present challenges for society
Generating shared prosperity for society will be a challenge. Robots and artificial intelligence will be more powerful than the industrial revolution because they replace not only routine, but also complex mental functions.
If handled well it may be the next best thing for civilisation and the UK has a strong history of inspiring leadership in machine learning (e.g. Alan Turing’s famous Turing Test of “can machines think?”). And with centres of excellence and high-profile companies including DeepMind and SwiftKey we are well placed to take a leading role.
But this utopia isn’t automatically guaranteed unless:
- New jobs are created offering greater variety
- Government continues to embrace investment, just as it does by providing access to open data and supporting start-ups with growth hubs. This approach will enable companies to thrive
- The education curriculum is advanced to ensure a stronger skills base. Skills such as mathematics and computer science should be particularly important with strong grounding in wider societal implications. And at the same time workers will need to take responsibility for ensuring they are digitally literate
- Workers must be confident interacting with machine learning, seeing it as an opportunity to improve the way they work rather than a threat that may replace them
- Society must also be happy interacting with machines as there are some genuine concerns. For some it could be considered impersonal (should an algorithm really predict how relevant an application is?). Other people may question if machines can accurately interpret nuances (can a CV Score inadvertently make the wrong decision when challenged with a unique CV? – something we take very seriously)
- We need to know who will be held accountable when machine learning ‘goes wrong’. There are many different approaches but perhaps the most pragmatic is the Bolam Test: whether a reasonable human professional would have acted in the same way
- The biggest threat may come from those who have the technology and so tax policy must develop so we don’t risk widening income distribution issues with concentrated wealth
- The best antidote to concentrated wealth will be competition. Therefore, the government must ensure monopolies cannot form and stifle innovation
- Privacy legislation must ensure consent and that we don’t provide inappropriate datasets that lead to bias
- Ethically, machine learning should also be acceptable. Do we want positive discrimination for those with a statistically higher chance of success?
At present the field of artificial intelligence is emerging so it can be shaped. However looking back, the latest digital revolution shows we can’t be complacent and we haven’t seen action from government largely because they want investment and tax receipts.
How can you extract value from artificial intelligence?
If you are an employee, becoming adept in machine learning will be a very valuable skill. There are Massive Open Online Courses (MOOCs) available with the aim of increasing knowledge in machine learning.
But whether you represent an organisation or yourself there are two common mistakes you should avoid:
- Thinking AI is futuristic. It is not, it’s already here
- Being fixated on ‘the next big thing’ instead of appreciating a subtle change can have a ripple effect. Before the Gutenberg Press, books were hand-written with beautiful calligraphy but access was limited. However the press helped spread mass culture, religion, science and gave us the Reformation.
So start cheap and humble. Focus on extracting insights from large volumes of data; and just like machines, learn from your mistakes and focus on the desired outcome.
Recruiting data scientists
The biggest challenge to starting an AI project will be hiring data scientists. These people are already in high demand and can command attractive packages.
We often find multi-nationals are an employer of choice for data scientists because they have the reputational pull, training, large datasets, significant resources, attractive problems to solve, and of course can pay higher salaries. This does make it harder for start-ups to attract and retain the brightest employees. Therefore IT contractors are a popular ‘fix’.
Finding the right talent is also being exacerbated as the talent is free to work or migrate anywhere with such strong global demand (sadly, at the time of writing this article, before Brexit, the UK hasn’t been the most popular destination).
More worrying is the lack of diversity in machine learning. We need to attract a broad range of people with different perspectives to encourage innovation and ensure we aren’t forming development ‘myopia’. Moreover, we shouldn’t have a narrow range of developers impacting such a broad range of people without reflecting the diverse users they serve.
Therefore, to meet the needs of our customers in filling their data scientist roles and ensuring diversity, Recruitment Genius will continue to advertise across a wide range of channels to attract applicants.
==
Please feel free to share this article and give your thoughts.
Finally, how can you benefit from AI?
As featured in
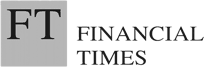
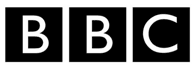
